AI in the lab
How scientists are leveraging artificial intelligence to unlock new solutions for growers everywhere
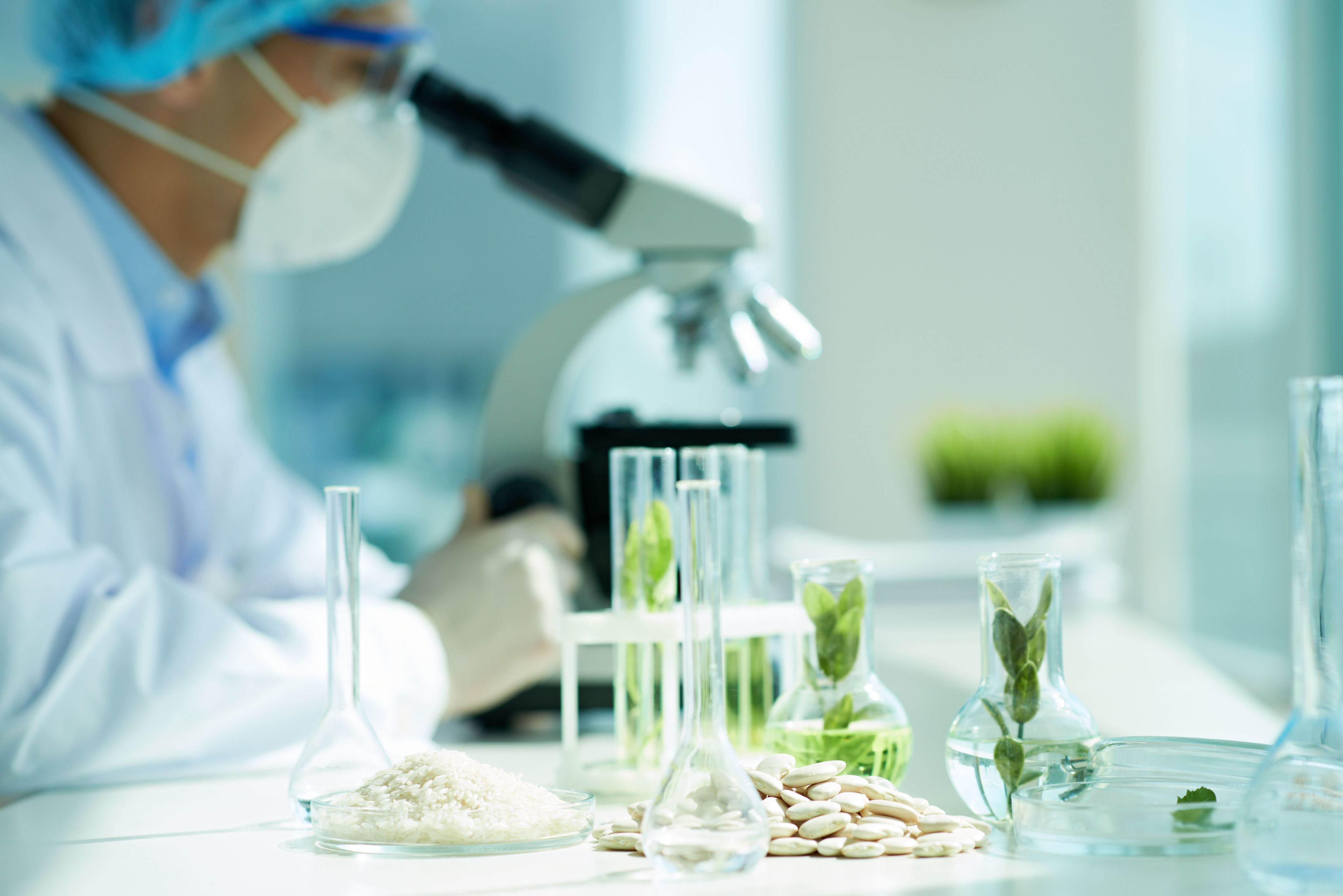
If there is one thing recent history has shown us, it’s how quickly technology can change the way we live. Just think of the breakthrough of the Internet or the generational changes made by the introduction of the first smartphone. Now we are going through another seismic technological shift driven by the innovative breakthroughs of artificial intelligence (AI).
Until recently, few of us may have heard of ChatGPT or Generative Artificial Intelligence (GenAI), but today, AI is part of our everyday lives, effortlessly generating things like text, presentations, social media posts and images.
And the world of research chemistry is no different. AI has been so influential that the 2024 Nobel Prize in Chemistry was awarded to the inventors of an AI model called AlphaFold that can predict the structures of proteins from genetic code. This is a breakthrough that can help accelerate drug discovery and our understanding of how diseases function.
The 2024 Nobel Prize in Chemistry was awarded to the inventors of an AI model called AlphaFold that predicts the structures of proteins from genetic code. Pictured: The predicted structure of the pesticidal crystal protein Cry6Aa Source: AlphaFold Protein Library.
The 2024 Nobel Prize in Chemistry was awarded to the inventors of an AI model called AlphaFold that predicts the structures of proteins from genetic code. Pictured: The predicted structure of the pesticidal crystal protein Cry6Aa Source: AlphaFold Protein Library.
In an era of complex and ever-evolving agricultural challenges, AI is helping chemists to create the next generation of sustainable products that can help protect crops from pests and diseases, in more efficient and effective ways.
In the lab, the process for designing and optimizing new crop protection products usually works on an iterative cycle – Design, Synthesis, Testing and Analysis, or DSTA for short.
Ed Emmett, Syngenta’s Head of Weed Control Chemistry, explains that: “AI touches every part of the DSTA cycle and at every stage we’re already using AI.”
To make sense of the impact that AI is having in this area of chemistry, let’s look at each stage of the process in more detail.
Design and discovery
Design is exactly what it sounds like – it’s the creation of a molecular blueprint. However, Chris Baker, Syngenta Head of Computational Chemistry Jealott's Hill, is quick to point out the sheer scale of the challenge involved in discovering a new molecule. He says: “The chemical space is enormous – there are more molecules we could make than there are stars in the universe, so where do we even start looking?”
This is one of the immediate advantages of AI – helping guide researchers to promising leads. Baker explains: “Computational algorithms can explore this space in a far more efficient way than humans can.”
Explaining another way that AI is a vital tool in the lab, Emmett says: “ChatGPT creates text in response to a prompt. In generative chemistry we can do the same thing to create the structure of molecules.”
With the right AI models and the right prompts, scientists can produce the structures of new molecular designs. These can then be evaluated, and scientists can even predict their properties all with digital tools. This makes pursuing promising ideas much faster and much more efficient.
These designed molecules need to meet a strict set of requirements around safety, efficacy, and sustainability. Emmett terms this ‘multi-parameter optimization’. To explain this way of working, he has a useful analogy: “If you are looking to move house there are so many different factors – you don’t do things in a linear fashion because that would just take too long.”
As a result, chemists work on what Emmett calls inverse design – rather than starting with a promising molecule, they begin with the parameters they want to meet as a way of informing design. All of this means you need to be able to predict these different factors – another way in which AI can be an effective ally.
Emmett sees AI as improving the ‘holistic quality' of the design phase. New molecules can be more specifically targeted and more effective, more cost-effective to make, and more sustainable all in one.
Just as with other generative AI models, much of the outcome depends on the quality of the prompt that you put into a generative design model. “You need to be able to accurately predict what’s going to happen, and which properties the molecule will have in the real world,” says Emmett.
Making a molecule is complex – not just because it must be effective, but it also must meet a long list of requirements covering issues from safety, cost-effectiveness and scalability to large-volume manufacturing.
It’s not surprising then that some models may need further refining. But even so, Emmett says that generative design “is a hot topic in the field and is already well established as a technology.”
Generative design in action: an AI model helps design a molecule optimized for multiple criteria.
Generative design in action: an AI model helps design a molecule optimized for multiple criteria.
Generative design in action: an AI model helps design a molecule optimized for multiple criteria.
Generative design in action: an AI model helps design a molecule optimized for multiple criteria.
Generative design in action: an AI model helps design a molecule optimized for multiple criteria.
Generative design in action: an AI model helps design a molecule optimized for multiple criteria.
Generative design in action: an AI model helps design a molecule optimized for multiple criteria.
Generative design in action: an AI model helps design a molecule optimized for multiple criteria.
Synthesis
The next stage of the cycle is synthesis – the point at which a potential molecule is made in a laboratory. Emmett says: “When people think about what chemistry is, they might think about mixing things together in a flask – AI helps answer the question of what will happen if we combine certain chemical components before physically carrying out a reaction.”
Artificial intelligence is helping chemists discover the next generation of sustainable products.
Artificial intelligence is helping chemists discover the next generation of sustainable products.
But that’s not all. When it comes to synthesizing a new chemical there are a lot of unknowns. With a highly detailed and sophisticated AI model scientists could make predictions on key questions about yield, safety, or even find ways of making chemical synthesis more sustainable.
However, synthesis is also the stage of the cycle where new challenges emerge.
Elizabeth Jones, Data for Design Lead at Syngenta, says: “Synthesis is a complex stage because models could suggest a molecular structure which isn’t synthetically feasible or would be far too costly.”
Guillaume Berthon, Syngenta Head of Digital Chemical Synthesis, agrees, highlighting that “currently around 30-40% of the molecules we intend to make are dropped along the way because of synthesis failures.”
To help solve this problem, Syngenta experts have partnered with technology leader IBM and MIT to use natural language processing (a method computer programs can use to understand human language) for what Jones calls ‘retrosynthesis prediction’.
This means making predictions about all the different ways a molecule could be assembled in a lab, based on data from all over the world. The models make predictions as a way of guiding the plan for the molecule that will be created.
The challenge is that models are only as good as the information on which they are trained, and public data may only cover which reactions have been successful as opposed to ones which didn’t work. Berthon agrees: “The areas of chemical space we are interested in are often poorly charted so public data cannot help synthesis very much.”
After all, even if a reaction was unsuccessful, scientists can learn a lot from what didn’t work and making the best models means utilizing the broadest range of data. “We always need to watch out for bias. If the quality of data isn’t good that can distort the models we work with,” says Emmett.
Automated image assessment generates more data to aid decision making.
Automated image assessment generates more data to aid decision making.
To create and train a model well requires data that is findable, accessible, interoperable and reusable – or FAIR for short. “FAIR data is vital to create and train models,” says Emmett, but “the digital infrastructure to support that needs to be developed further.” Key to that development is investing in getting the right data in the right format for machine learning.
This helps creates a virtual loop where high-quality data generates more relevant models that, in turn, inspire better synthetic procedures.
For Emmett the solution is to “leverage our data alongside publicly available data to make the best possible predictive models and it is these AI models that can enable valuable insight to be extracted.”
Testing
Next, the test phase. New crop protection molecules go through rigorous, stringent testing and here too AI plays an essential role.
Take plant biology. If a new molecule is developed to see if it is effective at controlling a disease on a plant, it must be tested out. Traditionally this might involve researchers in a greenhouse or out at a trial plot walking through rows of crops and making visual observations to judge its success.
But Emmett says: “Testing by eye to see if something is effective is subjective. AI imaging technology has the potential to give us far richer data. It’s not a surprise that everyone in research testing is exploring this opportunity.”
However, getting higher quality data is just one advantage offered by AI in this area.
Berthon explains: “The critical step is to make ‘inferences’ on this data. This is about answering a key question – ‘given my knowledge about the data generation process and the current data, what is the likelihood that my compound has the desired property?’
“Currently we do this manually, but AI will support us here too with probabilistic (so-called Bayesian) models which will ‘do the math’ for us and remove our biases,” he says.
Jones adds: “Rather than depend on subjective and qualitative human judgement, we do a lot of work with image recognition models that can assess the outcomes of assays and tests automatically.”
This automation is invaluable when trying to test out potential molecules at scale. Automated image recognition brings precision to testing leaves or plants in greenhouses. Baker adds: “We’re increasingly using this technology in the field, using drones to gather really valuable data on how compounds and molecules perform in the real world.”
Another way AI has changed the testing aspect of the cycle is through what he terms ‘active learning’. Previously, chemists would work on a compound and the most promising one would proceed through testing. However, with the help of AI, this process can be improved.
Baker says: “The AI algorithm can tell us which is the most important compound to test. This more proactive approach allows us to generate more high-quality testing data that we can then use to continue improving our AI models.”
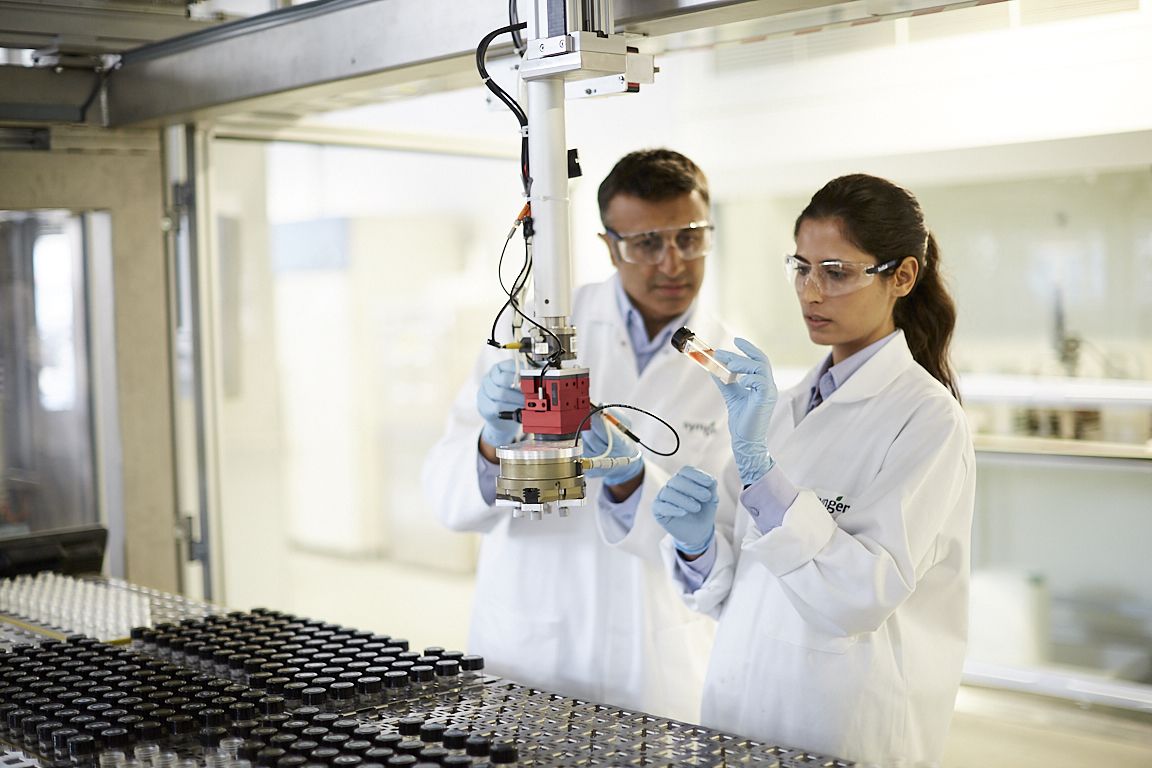
Analysis
Testing generates a lot of data and so here the analysis stage kicks into gear. “Good analysis of the data generated through testing allows for the creation of the best prompts for the next GenAI design step,” says Emmett.
Image recognition models can assess the outcomes of assays and tests automatically. Pictured: Image analysis of a herbicide assay.
Image recognition models can assess the outcomes of assays and tests automatically. Pictured: Image analysis of a herbicide assay.
As a result, analysis and design go hand in hand, because, as Emmett says: “We work in an iterative fashion where good analysis informs good design, thereby allowing us to reach our desired outcome as efficiently as possible.”
AI algorithms can process and analyze large datasets to identify patterns, trends, and correlations that might be too complex for traditional statistical methods and for humans to process.
Learning from these patterns, AI can then make accurate predictions about the properties of molecules yet to be made, such as their efficacy or safety. All of this can further help reduce experimental costs and accelerate the discovery process.
“The better the analysis, the fewer design, synthesis, test, analysis cycles you need to go through,” Emmett says.
Elizabeth Jones, Data for Design Lead and Chris Baker, Head of Computational Chemistry Jealott's Hill.
Elizabeth Jones, Data for Design Lead and Chris Baker, Head of Computational Chemistry Jealott's Hill.
AI has the potential to make a seismic impact across the DSTA cycle, unlocking a vast amount of insight-rich data that can help drive high-quality innovation, more quickly and more sustainably than before.
As technology advances, scientific research unlocks more data than ever. “AI helps us make sense of the sheer scale of information – making it an essential tool for scientists to help farmers everywhere protect their crops,” Emmett says.
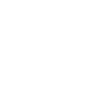